Heart Disease Progression
Heart disease progression refers to the process by which heart disease develops and worsens over time. It often starts quietly, progressing from high blood pressure to clogged coronary arteries with few obvious symptoms. Over time, heart failure can lead to dysfunction of other organs due to inefficient pumping. Understanding each stage of heart disease can help you work with your doctor to slow its progression or, better yet, even reverse it.
Stages of Heart Disease Progression
Stage A
People with stage A heart failure do not yet have issues with their heart’s pumping activity but have a high risk of developing heart failure due to related conditions, such as chronic high blood pressure, diabetes, and coronary artery disease. At this stage, treatment should focus on managing risk factors.
Stage B
At this stage, structural heart disease develops, such as reduced heart pumping, which can lead to an enlarged left ventricle. Most people at stage B still do not show symptoms of heart failure. A doctor may prescribe medication at this stage for the management and prevention of future issues.
Stage C
People at this stage will show symptoms of heart failure linked to underlying structural heart disease, including fatigue or breathlessness. These symptoms usually occur due to problems with the squeezing function of the left ventricle or the pumping chamber of the heart. Common symptoms include fatigue, breathlessness, difficulty breathing, lower extremity edema (swelling), difficulties laying flat, rapid weight gain, and abdominal distension.
Stage D
This is the most advanced stage of heart disease. At this stage, heart failure symptoms become severe and significantly impact a person’s quality of life. Despite receiving optimal medical therapy, symptoms persist. Advanced treatments, such as mechanical circulatory support, heart transplant, or palliative care, may be considered.
Heart disease progression is a complex process that involves various stages. Each stage has its own characteristics and requires different treatment approaches. Early detection and appropriate management can slow down the progression of the disease and improve the quality of life for patients.
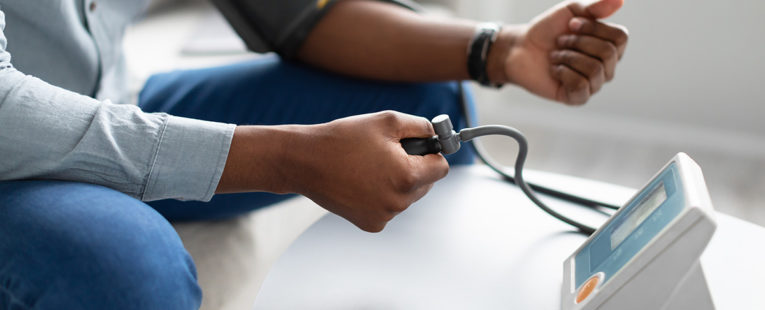
What are the predictive models for heart disease progression?
Predictive models of heart disease progression are computational tools that use various patient characteristics to estimate the probability of outcomes over a certain period of time. These models, often based on machine learning algorithms, have shown significant promise in enhancing heart disease prediction precision and effectiveness.
Types of Predictive Models
Machine Learning Models
Machine learning models have been widely used in predicting heart disease. These models can handle large amounts of data and can learn from it to make predictions. Some of the commonly used machine learning models include:
Random Forest
Decision Tree Classifier
Decision Tree Classifier is another machine learning model used in heart disease prediction. It is a type of flowchart-like structure where each internal node denotes a test on an attribute, each branch represents the outcome of a test, and each leaf node holds a class label.
Multilayer Perceptron
Multilayer Perceptron is a type of artificial neural network model that is used for heart disease prediction. It consists of multiple layers of nodes in a directed graph, with each layer fully connected to the next one.
XGBoost
Random Forest is a popular machine learning algorithm used in heart disease prediction. It is an ensemble learning method that operates by constructing multiple decision trees and outputs the class that is the mode of the classes output by individual trees.
XGBoost is a decision-tree-based ensemble machine learning algorithm that uses a gradient boosting framework. It has been used in the prediction of heart disease due to its effectiveness and efficiency.
Cloud-Based Models
Cloud-based prediction models for cardiovascular disease integrate machine learning and feature selection techniques. These models are implemented in a cloud computing environment, enhancing the scalability of cardiac prediction systems.
Predictive models of heart disease progression have the potential to revolutionize the way we understand, diagnose, and treat heart disease. By leveraging advanced data analysis techniques and machine learning algorithms, these models can provide valuable insights for improving performance in higher prediction rates, recall precision rates, and management of cardiovascular health.
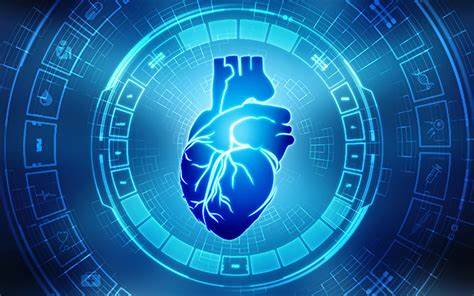
Benefits of Machine learning (ML)
Machine learning (ML) offers several benefits in the progression of heart disease. Here are some key advantages:
Early Prediction and Intervention
Machine learning models can analyze vast datasets and discern intricate patterns, which can revolutionize the prediction of cardiovascular disease. Early prediction and intervention emerge as pivotal strategies in mitigating the burden of heart disease. Timely identification of individuals at risk allows for targeted preventive measures, fostering a paradigm shift from reactive to proactive healthcare.
Unveiling Risk Factors
Machine learning can help unveil risk factors from health records. Key findings highlight the significance of genetic predispositions, lifestyle choices, and clinical markers as influential contributors to heart disease development. The integration of these factors into machine learning models demonstrates notable accuracy in preemptive risk assessment.
Improved Accuracy and Generalizability
Machine learning-based models have been used to predict cardiovascular events with improved accuracy and generalizability compared to traditional risk predictors. These models provide a powerful tool for learning complex relationships between the risk predictors and outcomes from a representative sample of the patients.
Holistic Picture of Heart Failure
Using machine learning in heart failure research has the potential to combine information from different sources, subjects, and scales to provide a more holistic picture of the failing heart and point toward new treatment strategies.
Personalized Healthcare Strategies
The implications of machine learning in heart disease are profound, offering potential advancements in preventive healthcare strategies, personalized interventions, and resource allocation for populations at heightened cardiovascular risk.
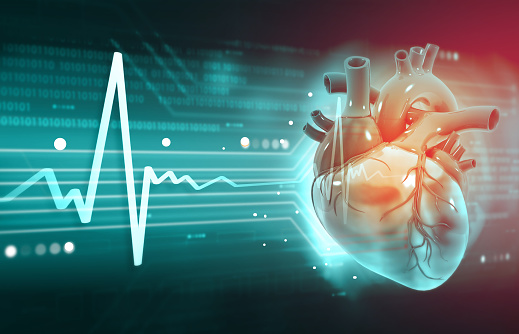
Pioneering AI-Driven Heart Disease Progression
At AIMPH, we’re revolutionizing healthcare with our AI-driven Multi-physics simulation and optimization. We’re harnessing the power of artificial intelligence to transform the way heart failure and disease is diagnosed, particularly in the Middle East. Our mission is to implement cutting-edge machine learning models for the early diagnosis of heart failure and heart disease progression.
Collaboration and Partnership
We believe in the power of collaboration. At AIMPH, we’re open to partnerships with other companies and healthcare professionals. Together, we can expedite implementation, close healthcare gaps, and pave the way for personalized medicine.
Our AI techniques can eliminate the need for costly and extensive clinical and laboratory investigations. Also, reducing the financial burden on patients and the healthcare system. Moreover, our models can provide healthcare professionals with valuable insights, enabling them to make informed decisions about patient care.
Join us on our journey as we pioneer the future of healthcare with AI-driven diagnostics. Together, we can make a difference in the lives of millions of people suffering from heart failure.
Welcome to AIMPH, where AI meets heart health.
Share AIMPH with your friends and collogues
AIMPH is your partner in Oman and Middle East for your AI-driven projects. Let’s connect your strategy and business with codes. You can share this post kindly if it’s useful for your fiends and collogues.
Predictive modeling of heart disease progression
Heart Disease Progression Heart disease progression refers to the process by which heart disease dev…
Heart Failure’s Predictive Models
Predictive Models for Heart Failure There are several predictive models for heart failure, each with…
Predictive hospital resource allocation in Oman
Predictive Analytics for Hospital Resource Allocation Predictive analytics in healthcare is a p…
Clinical trial research optimization for Oman healthcare
Clinical Trial Research Optimization Clinical Trial Research Optimization involves strategicall…
Cryptocurrencies sentiment analysis with AI
Cryptocurrencies Sentiment Analysis Analysis of cryptocurrency sentiment Artificial intelligence (AI…
Fault detection in mechanical systems with powerful AI
Fault detection in mechanical systems Utilizing powerful AI technology for fault detection in mechan…