Predictive Models for Heart Failure
There are several predictive models for heart failure, each with its own strengths and limitations. Each of these models uses different sets of variables and techniques to predict heart failur. Their effectiveness can vary depending on the specific circumstances and data available. It’s important to consult with healthcare professionals to understand which model might be most appropriate for a given situation. First of all what is the heart failure?
What is exactly heart failure?
Heart failure, sometimes referred to as congestive heart failure, is a condition where the heart can’t pump blood as well as it should. This can lead to a variety of symptoms and complications. Here are some key points:
- Causes: Heart failure often occurs when certain heart conditions gradually leave the heart too weak or stiff to fill and pump blood properly. These conditions include narrowed arteries in the heart and high blood pressure.
- Symptoms: Symptoms may develop slowly or start suddenly. They may include shortness of breath with activity or when lying down, fatigue and weakness, swelling in the legs, ankles and feet, rapid or irregular heartbeat, reduced ability to exercise, and others.
- Complications: Over time, the heart tries to compensate by enlarging, developing more muscle mass, and pumping faster. However, these compensations can lead to further complications.
- Treatment: Proper treatment may improve the symptoms of heart failure and may help some people live longer. Lifestyle changes such as weight loss, exercise, reduced salt intake, and stress management can improve quality of life. In severe cases, some people may need a heart transplant or a device to help the heart pump blood.
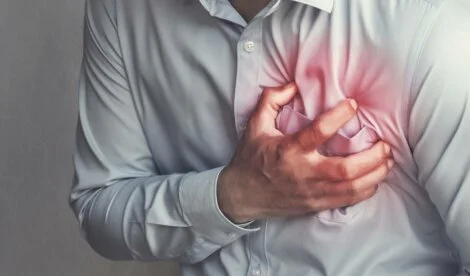
What are the predictive models for heart failure?
- Machine Learning Models: Machine learning methods have been compared with traditional models for predicting heart failure outcomes. These models can predict key patient outcomes in heart failure with modest discrimination and accuracy.
- Lifetime Risk Prediction Models: A Northwestern Medicine study has derived and validated the first set of risk prediction models for lifetime heart failure risk. By answering a few basic questions at the doctor, you can receive an estimate of whether you’ll experience heart failure in the next 30 years.
- Traditional Models: Traditional models like the ADHERE and the Seattle Heart Failure model have been developed to estimate the risk of death for patients with heart failure.
Machine Learning for heart failure
Machine learning models for heart failure prediction typically involve the use of algorithms that can learn from data. Also, make decisions or predictions based on data. Here’s a more detailed explanation:
- Data Collection: The first step in building a machine learning model is data collection. In the case of heart failure prediction, this could involve collecting electronic medical records of patients which quantify symptoms, body features, and clinical laboratory test values.
- Feature Selection: The next step is feature selection, where the most relevant features (or risk factors) that may lead to heart failure are identified. Also, ML can be used to detect these important clinical features. For instance, a study found that serum creatinine and ejection fraction were the two most relevant features for predicting survival of patients with heart failure.
- Model Training: Once the features have been selected, the machine learning model is trained using a portion of the collected data. During this process, the model learns to recognize patterns and correlations in the data that are indicative of heart failure.
- Model Testing: The model is then tested on a separate portion of the data (not used in training) to evaluate its performance. The goal is to ensure that the model can generalize well to new, unseen data.
- Prediction: Once the model has been trained and tested, it can be used to predict the survival of patients with heart failure from their data.
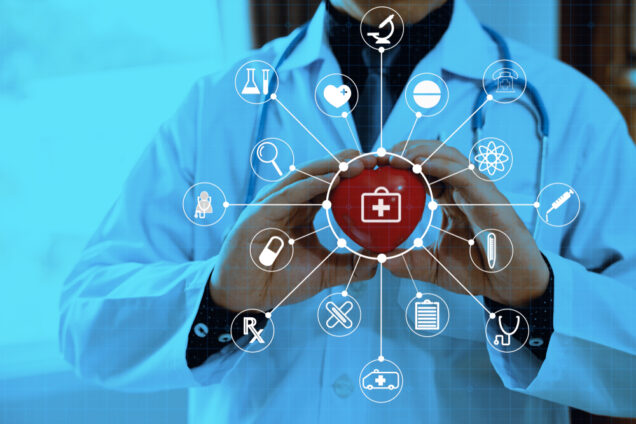
Benefits of Machine learning (ML) in the early diagnosis of heart failure
- Early Detection: ML can help in the early detection of heart disease, which is crucial for taking necessary steps at the right time. It can identify intricate associations and detect early-stage heart disease.
- Efficiency and Accuracy: ML algorithms enhance the accuracy and efficiency of heart failure diagnosis. They can handle large and complex datasets, and do not require predictors.
- Risk Factor Identification: ML can confirm major risk factors for heart failure.
- Cost-Effective: By developing evidence-based clinical guidelines and management algorithms, ML techniques can eliminate the need for costly and extensive clinical and laboratory investigations, reducing the financial burden on patients and the healthcare system.
- Personalized Medicine: ML pave the way for personalized medicine by identifying subtypes of heart failure beyond those that are currently recognized and improving risk prediction.
- Healthcare Gaps: ML can expedite implementation and close healthcare gaps in learning healthcare systems.
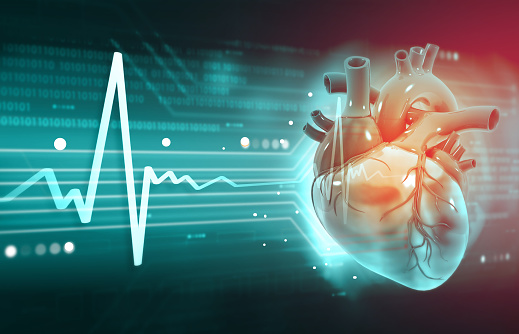
Pioneering AI-Driven Heart Failure Diagnosis
At AIMPH, we’re revolutionizing healthcare with our AI-driven Multi-physics simulation and optimization. We’re harnessing the power of artificial intelligence to transform the way heart failure is diagnosed, particularly in the Middle East. Our mission is to implement cutting-edge machine learning models for the early diagnosis of heart failure.
Collaboration and Partnership
We believe in the power of collaboration. At AIMPH, we’re open to partnerships with other companies and healthcare professionals. Together, we can expedite implementation, close healthcare gaps, and pave the way for personalized medicine.
Our AI techniques can eliminate the need for costly and extensive clinical and laboratory investigations. Also, reducing the financial burden on patients and the healthcare system. Moreover, our models can provide healthcare professionals with valuable insights, enabling them to make informed decisions about patient care.
Join us on our journey as we pioneer the future of healthcare with AI-driven diagnostics. Together, we can make a difference in the lives of millions of people suffering from heart failure.
Welcome to AIMPH, where AI meets heart health.
Share AIMPH with your friends and collogues
AIMPH is your partner in Oman and Middle East for your AI-driven projects. Let’s connect your strategy and business with codes. You can share this post kindly if it’s useful for your fiends and collogues.
Predictive modeling of heart disease progression
Heart Disease Progression Heart disease progression refers to the process by which heart disease dev…
Heart Failure’s Predictive Models
Predictive Models for Heart Failure There are several predictive models for heart failure, each with…
Predictive hospital resource allocation in Oman
Predictive Analytics for Hospital Resource Allocation Predictive analytics in healthcare is a p…
Clinical trial research optimization for Oman healthcare
Clinical Trial Research Optimization Clinical Trial Research Optimization involves strategicall…
Cryptocurrencies sentiment analysis with AI
Cryptocurrencies Sentiment Analysis Analysis of cryptocurrency sentiment Artificial intelligence (AI…
Fault detection in mechanical systems with powerful AI
Fault detection in mechanical systems Utilizing powerful AI technology for fault detection in mechan…